with Dr. Paul Remo Wagner from Matrisk
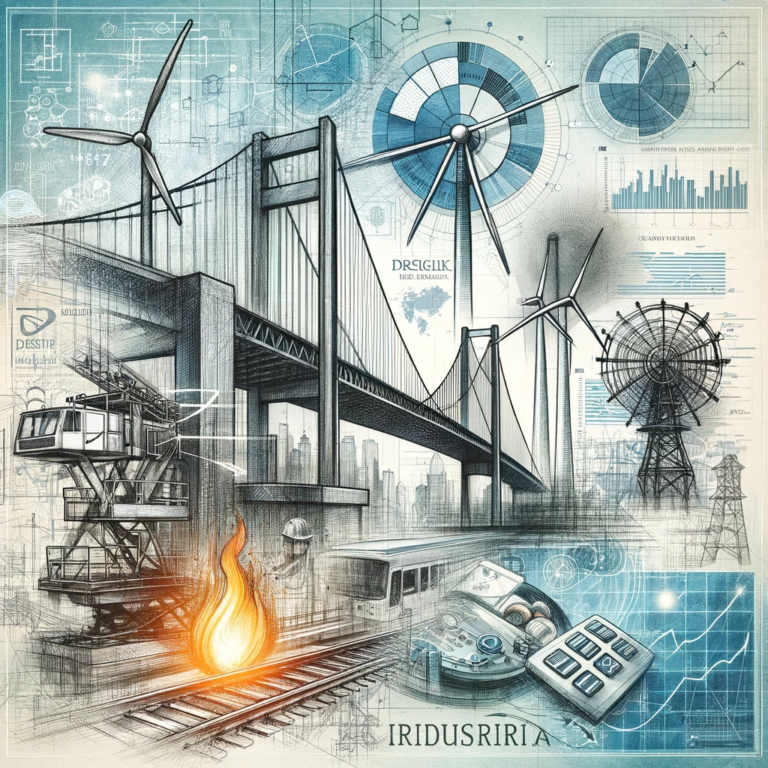
Summary
In this episode of “Concretely,” host Johannes Lohner interviews Dr. Paul-Remo Wagner from Matrisk, focusing on quantitative risk analysis in engineering. Dr. Wagner discusses Matrisk’s approach to integrating quantitative risk analysis in assessing and mitigating potential negative impacts of infrastructure systems, including economic costs and human safety considerations. The conversation also explores into the challenges of combining expert knowledge with data, the importance of probabilistic models in engineering, and the process of model calibration and validation in risk analysis projects.
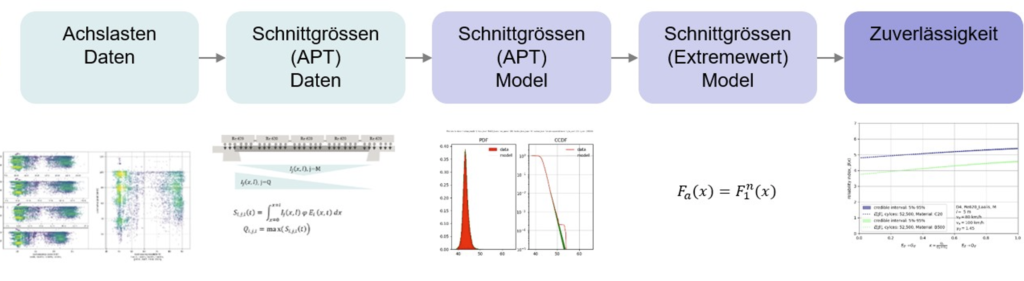
Wonder how to use quantitative risk analysis in construction?
Listen to the transcript or read the white paper. Comment below
Transcript
[responsivevoice buttontext=”Play Transcript”]
Johannes: Welcome to Concretely.
I am your host, Johannes Lohner, and I talk with experts from different disciplines about the preservation of our structures.
Today we are discussing quantitative risk analysis. We’ll be talking with Doctor Paul-Remo Wagner from the company Matrisk. Remo is an expert in risk modeling. Matrisk was founded in 2004 by four partners, three of whom hold professorships at technical universities in Europe. Welcome, Paul.
Paul-Remo: Hello, yes. Thank you very much for the invitation. I’m pleased to be here talking with you today.
Johannes: Can you briefly explain what Matrisk offers its customers?
Paul-Remo: Yes, I think it’s difficult to summarize it briefly, but I think one way to put it is that our focus is on quantitative risk analysis. This means concretely that when we create risk analyses – usually when it comes to assessing the mostly negative impacts of our clients’ systems – we always try to quantify it, which means attaching numbers to the assessment. Numbers usually in the form of costs per year, which is the typical economic approach: what does it cost us to operate a railway line or bridge, while considering that this bridge and this structure can fail, need maintenance, and so on. And also in certain systems, in certain cases, it’s also about considering the fatality of people, users of these structures, in this whole analysis. So the important thing, why quantitative things are important at all. Maybe that’s still somehow important. Because there are the qualitative approaches where one tries to avoid these negative consequences, so the failure of some things. So you set measures to circumvent such things. With quantitative matters, it’s about recognizing that these things are unavoidable, and then trying to say: Okay, if these things are unavoidable, then we first make sure that they occur as rarely as possible, and then we also say how rarely they occur to be able to classify that.
Johannes: Is that why your homepage states that you are Bayesians, from the Bayesian theorem, or where does that come from?
Paul-Remo: It’s on there because we work with Bayesian methods, which come from the Bayesian theorem, yes, but there are also Bayesian statistical methods. And they are extremely well suited for combining expert knowledge, which is extremely common in engineering, with data. So, combining data with expert knowledge, that’s one of the things for which the Bayesian approach is really well suited.
Johannes: Maybe a follow-up question, do you sometimes encounter conflicts because experience counts for so much, and when you then come with data and statistics and perhaps then show that in some cases experiences are wrong or lie differently? Are there conflicts with engineers, or do you sometimes have problems convincing them?
Paul-Remo: I wouldn’t call them conflicts right now, I would say differences of opinion or understanding difficulties. That’s what I would say is most common in the relationship. Many things play into this, but also this approach of treating problems, let’s say, probabilistically. This means not only calculating with fixed values and avoiding something from happening, but recognizing that something can happen and minimizing the probability of occurrence. That’s an approach that many engineers struggle with, I would say. But understandably, of course, because in many engineering applications, these simplifications, which are in reality deterministic treatments, must be made to make the problems somehow manageable. But sure, sometimes we would wish there were a bit more understanding for a broadening of this perspective. In certain cases, special cases where then such a deterministic view or purely expertise-driven view without considering new findings from data and so on. Where we would then wish that these might be taken up more, that maybe, yes.
Johannes: How is it that you mentioned earlier that you also quantify in the case of people and fatalities. How can one quantify a fatality or human life in such analyses?
Paul-Remo: Yes, that’s a very difficult question. We are, on the one hand, of course, the wrong people. I think the debate about quantifying people, you could discuss that with philosophers, on an ethical level, for years. With us, it’s always different from case to case. I can’t name sums now, that would be firstly unprofessional and secondly also wrong. But when you approach the debate somehow and think about it, then you also have to be aware that we always take certain risks. We take certain risks without really thinking about how big the risks really are. So, we all get in the car, travel by train, airplane, and we know exactly that these things are not 100% safe, that something can always happen. But because of that, one is then never aware that even a safe structure has a certain probability of failure. And if this probability of failure now lies very low, say every
1000 years, then we just accept that because the alternative would be to dimension these structures so that it causes societal problems. So if you design a bridge so that it absolutely cannot collapse, under no conceivable load, then we can build one bridge per year worldwide, and that’s it. So, you have to find some trade-off somewhere, and human life plays into that as well.
Johannes: Currently, this is probably going to be an issue in the coming years, as the infrastructure in Europe and the US, in industrialized countries, gets old, right?
Paul-Remo: Absolutely, absolutely, so that is certainly an important topic.
Johannes: Maybe you can name a few examples, you’ve already mentioned the bridge example, where you’ve brought added value to your customers?
Paul-Remo: Yes, gladly, so applications of these risk analyses. So, one example I’ve already mentioned, right? We had a really cool project with the SBB, where, based on wheel load measurements from various railroad cars at different locations in Switzerland, we received data from the SBB. And we were able to build a probabilistic load model with this data, meaning a distribution of the loads that typically occur on such a bridge. And then we took this load model and, based on a simplified model for various bridges in Switzerland, looked at how safe the SBB’s bridge portfolio is in terms of failure probability with these loads. And that was quite interesting to see. First, because there are even reserves in certain types of bridges, where the traffic loads, of course, with more detailed analysis, but based on these first steps, it would be possible to even increase the traffic loads. And to allow a higher utilization on these bridges. And that was possible with this populist analysis. That’s about bridges.
And then we also had a cool project, together with the Association of Cantonal Fire Insurances (VKF), which is still ongoing. That is also just now in the hot phase, where it’s about unifying fire protection in Switzerland, which is very differently regulated at the cantonal level. So not only us, it’s a large consortium, but we are the experts for the risk-based approach, and it’s also about determining. So, first of all, based on data, how often does it actually burn and where does it burn in what kind of buildings. And afterwards, when we know how often it burns somewhere, how efficient are certain fire protection measures. So we try to assess how efficient certain measures, in terms of containing fire development, actually are. And that also in the form of quantitative risk reduction. That means if you use a measure, by how much does the risk reduce damage. And if you do that, then you have a tool in hand to evaluate afterwards how much such a measure may cost. And that is a mega interesting case because in fire protection there are always very many different measures, but they have never been really ranked in their efficiency. And that would be an outcome that we are working on very intensively and that would offer great added value, not only for the VKF but I think also for fire protection in Switzerland as a whole.
Yes, and then another third project, maybe more towards infrastructure again. We have a large project going on where it’s about developing software for probabilistic digital twins for offshore wind farms, not so much in Switzerland, we don’t have that much there, but in the North Sea/Baltic Sea, we are developing software for probabilistic digital twins. It’s about modeling structures. But not deterministically, but probabilistically, to map the uncertainties in these structures and coupled with optimal risk-based inspection plans. This means how often should these structures be inspected. That means where exactly do you look and inspect. And yes, we are also right on that, and the goal is of course to enable a cheaper operation on the one hand, but also to possibly extend the lifetime of these wind farms, depending on the loads that have already occurred.
Johannes: Those are very exciting topics.
Paul-Remo: Yes, I think so too.
Johannes: Can you explain step by step to the listeners and me, how a project on quantitative risk analysis runs, if one can say that in general?
Paul-Remo: Well, it’s not that simple. Generalizing the approach is difficult, but I think the first step, which is actually common to every project, is the most important one – that we work together with the clients, who are naturally the experts in their various applications, relative to us. We are risk experts, but we are not experts in fire protection, bridge construction, or offshore wind. Therefore, it is always important for us that they share the information they have. This is especially difficult because probabilistic approaches or quantitative risk matters are not the daily bread of engineers in many cases, and it is often extremely important, and usually important from the beginning, to go in and explain what we do. And then also the knowledge that engineers often possess, but are not aware that they have a lot of data for certain things or are not aware that they are capable of modeling these phenomena probabilistically themselves after a short introduction, let’s say. Therefore, scouting, I would say, is the first and important step. For example, in the case of the SBB project, we were able to develop the load model to map the traffic loads on the bridges, and we did not start our own data collection for this. Rather, it was actually the case that the SBB operates a wheel load measurement system on their network anyway, but for entirely different reasons. It’s about tracking the trains when they are too heavy for certain sections of the route. And they also collect all these data, which is great and not taken for granted. Often data is still somewhat seen as a necessary evil that you have to delete from time to time, but it was really great that they didn’t do that and made it available to us. And we had a treasure trove of data that we could then use, and that was great.
Johannes: Those were the loads of the trains that go over the bridge, right?
Paul-Remo: Exactly, the data they collected were wheel load measurements, not just for bridges but also for other critical sections of track where a certain maximum load is permissible. They have a safety system where they say from a certain load, a train cannot continue. And they have data from every train that passes over, which were over 2 million wheel load measurements.
Johannes: Not bad, okay…
Paul-Remo: Yes, and then the next step, after you have worked out the problem. This often comes out of such conversations, that you are not initially so aware of what the problem, the problem statement, exactly is. For us, model development usually comes next. That means we develop a model for the specific problem, which in this SBB case is a load model, based on these data (a purely data-based load model), coupled with the simplified structural model of the bridges, and then we can start our analysis. What might also be important in model development, I’ve already mentioned, with the probabilistic models. In model development, it is often the case, not just with us as a company, but actually often in engineering, that we don’t know certain input sizes in models so precisely. So, these are the traffic loads here, the perfect case. We don’t know exactly how heavy each train is that goes over the bridge. And with probabilistic models, you can take that into account. Instead of simply modeling an extreme case of the train, which is the typical approach, you model this train as uncertain, or ‘uncertain’ is actually the right translation of ‘uncertainty’, you model these sizes simply as not specifically known and then look at how these uncertainties propagate through the models, i.e., what effects this then has on the stresses in the bridge when the traffic loads are considered uncertain. And then take these uncertainties into account, not just on the impact side, which are the loads in this case, but also on the resistance side. We know, for example, from materials science that the yield point of steel, for example. Steel is relatively safe, but there are also uncertainties in the material models. But concrete, for example, the resistance of concrete is not always the same, as they say, it varies extremely. And this can be taken into account with probabilistic models. And the result of such a model is then not that a bridge fails or does not fail if it is incorrectly dimensioned or if heavy loads drive over it, but the result is a probability of failure. So we can then say with a certain probability the bridge fails and can then cover much broader cases than just looking at an extreme value.
Exactly that to model development, and then it is also important to calibrate the model. So, it is important to work with data to either model input parameters directly or, the classic calibration goes so far that one looks at the outputs of the model and takes other data to match the outputs with the data. There are various approaches for this. You can do this probabilistically or deterministically. There
are many possibilities and also here, as we have already briefly mentioned before, the Bayesian methods are also such an important tool for model calibration. And model calibration is a classic case where you work with expert knowledge, that is, with existing knowledge about uncertainties, which are then often known to engineers. So, when you then ask them, you have a material strength, do you know exactly how big it is? They often don’t know that. But if you ask them, can you give us a range, i.e., minimum and maximum values, that is very often known. And we can then use that to model it probabilistically. And then also use that to calibrate it.
Johannes: So you calibrate the model afterwards and adjust it so that it meets the expectations and results. To me, it sounds a bit like supervised learning from the machine learning area. Can you compare it?
Paul-Remo: Yes, certainly. So, ‘supervised learning’ is basically the ‘training’ actually. The ‘training’ of models in machine learning models is basically nothing else. There, you take the inputs into the model and the outputs, in the case of machine learning then the ‘labeled’ input data. Then the model is trained, and that means nothing else than that the parameters of these models, which often have many, many more parameters than our models, so much more, hundreds of thousands and so on, are adjusted and trained, or calibrated, you can also say. But the main difference is actually that our models are often lower dimensional, so have many fewer parameters, but are therefore much more expensive, in the sense of, if you have a structural model of a bridge, they are very computationally intensive. It can be, depending on what kind of model you have, that such a finite element model (FE model) of a bridge takes hours or days to calculate. For this, there are then approaches to model even such expensive models. These are also things we do. For example, keyword surrogate modeling, ‘surrogate model’ in English.
So then, when the model is calibrated, it is also very important that we go back to our customers with the model, with the calibrated model. And take another step to pick up their knowledge again and also to gain some credibility for the model. Because ultimately, models are just models. They are never more than models. They have their use in that area, but do not correspond to reality. And therefore, it is extremely important that you go in there with the customers together again and look in detail where there might be misunderstandings or not, or where value has already been brought to the customer here. And therefore, this iteration step is something very, very important.
Johannes: Ok, so those were all the steps?
Paul-Remo: Yes, shall I summarize it again?
Johannes: Maybe I’ll summarize it, from my understanding: So first the scouting, it’s about collecting data. I imagine that to be very complex because the customer doesn’t always know exactly what data is necessary and what is available at all. The second step is model development. Then comes model calibration, where everything is put together again and checked that the model works correctly and is adapted to the data. And then the last step, going back to the customer iteratively, reconciling again, discussing and explaining. Is that correct?
Paul-Remo: Exactly, and then at the end, of course, generating the results. That is then the actual goal: the predictions or the recommendations for action. That is where the model really comes into play. It allows a quantitative risk analysis to be done and to say: Ok, based on the model, it makes the most sense to do this or that and to refrain from doing this or that.
Johannes: Ok, maybe fundamentally. Is a model always needed for quantitative risk analysis, or is sometimes just analyzing the data sufficient?
Paul-Remo: Well, fundamentally it depends on what one means by a model. There are purely data-driven models, where you don’t go through the entire process of model building, but simply receive data and develop a model based on it.
However, there are some problems with our applications. The first is certainly that we often simply do not have data or not as much data as we need. This is changing at the moment, so there is a clear trend in this direction. Awareness of the value of data is rising. But it is not really established for all applications first, and second, it is not possible everywhere. For example, with a bridge, to come back to bridges: If you have a bridge, which is a single structure, it is difficult, depending on the question, to do it purely data-based. That doesn’t mean that it can’t be done probabilistically, but purely data-based is difficult. That’s why model building, as we understand it, is very important. The second thing for purely data-based approaches is that we often work with so-called tail events. So it’s about rare events, like high loads, low material strengths. That is the area where we do risk analyses, and purely data-based it just takes a very long time to statistically capture these rare events. Therefore, some form of model building is essential in most cases.
Johannes: What are the biggest challenges in the projects?
Paul-Remo: Yes, well, I believe the biggest challenges in my experience are not necessarily technical. Not the work we do per se, but communication, both at the start of a project, in this exploration phase, where it’s about understanding or conveying where we can provide added value. And then again in the communication of the results. On the one hand, because in the validation process much more knowledge can be retrieved when the customer is involved. I’ve said this before: We are not experts in the individual fields we serve. We are risk experts and therefore heavily reliant on the expertise of the customers in the individual areas. And there are often difficulties in terms of communication and choice of words, and how to explain what we actually do. These are the challenges.
Johannes: Thank you for listening. Please share your opinion in the comment section of the episode on my homepage www.concrete-ly.com. You are also very welcome to leave personal experiences or complaints. Please “like” and share the podcast with your friends and colleagues, also via Spotify or Apple Music. By the way, the episodes are also translated into English on the homepage.
[/responsivevoice]
Literature
- Book: Melchers R. E and Beck A. T. (2017), Structural Reliability Analysis and Prediction, 3rd Edition
- Book: Gelman, A. et al. (2021), Bayesian Data Analysis, 3rd Edition
- ETH lecture notes: Structural Reliability and Risk Analysis
- Online handbook: handbook.reliability.space (especially part 6)
White Paper on Quantitative Risk Analysis in Construction
Key Take-Aways:· Balancing Safety and Feasibility: The critical importance of finding a trade-off between ensuring absolute safety and maintaining practical feasibility in construction, highlighting the need for balanced design choices to manage resources effectively. · Innovative Approaches for Aging Infrastructure: Insights into tackling the challenges posed by aging infrastructure in industrialized countries through advanced quantitative risk analysis, emphasizing the growing necessity of such methods for maintaining structural integrity and safety. · Real-World Applications and Impact: Showcasing the benefits of quantitative risk analysis through detailed case studies, including projects on bridge load capacity, fire protection efficiency, and offshore wind farm maintenance, demonstrating the practical impact and added value of this approach in diverse engineering scenarios. |
Introduction
This white paper synthesizes the insights from the “Concretely” podcast episode titled “Quantitative Risk Analysis in Construction,” featuring an interview with Dr. Paul-Remo Wagner from Matrisk. The episode explores the integration of quantitative risk analysis in assessing and mitigating potential negative impacts on infrastructure systems, with a focus on economic costs and human safety.
Understanding Quantitative Risk Analysis
Quantitative risk analysis in construction involves assessing the probability of failure in structures and finding a balance between absolute safety and practical feasibility. Dr. Wagner points out that clients sometimes find probabilistic concepts difficult to understand. They often prefer more traditional approaches that ignore uncertainties, rather than acknowledging the probabilistic nature of accidents and managing their occurrence accordingly.
Overcoming this challenge involves educating clients on the importance and advantages of considering probabilities and preparing for various outcomes, instead of focusing solely on accident prevention. Dr. Wagner emphasizes the necessity of a trade-off, as overly cautious designs contradict the goal of efficient societal spending that is of utmost importance in the face of limited resources.
Key Points:· Probability of failure is a critical metric in construction risk analysis. · Designing for excessive safety is impractical and resource intensive. · A balance must be found between safety and feasibility. |
Aging Infrastructure: A Growing Concern
The discussion highlights the aging infrastructure in Europe and the US, pointing out the increasing importance of effective risk analysis in these regions.
Key Points:· The aging infrastructure in industrialized countries poses new challenges. · Effective risk analysis is crucial for maintaining safety and functionality. |
Case Studies: Application of Quantitative Risk Analysis
Dr. Wagner provides examples of Matrisk’s application of risk analysis in various projects, showcasing the practical implications and benefits of their approach.
I. Case 1: SBB Project
- Collaborated with the Swiss Federal Railways (SBB).
- Developed a probabilistic load model for bridges based on wheel load measurements.
- Result: Identified potential for increased traffic loads on certain bridges.
II. Case 2: Fire Protection Regulation Project
- Partnership with the Association of Cantonal Fire Insurances (VKF).
- Focused on unifying fire protection regulation across Switzerland.
- Evaluated the efficiency of fire protection measures in terms of quantitative risk reduction.
- Result: Ranked effectiveness of fire protection and allow for cost-effective safety improvements.
III. Case 3: Offshore Wind Farms
- Developed software for probabilistic digital twins for offshore wind farms.
- Implemented tools for risk-based inspection planning considering uncertainties.
- Result: Optimized inspection plans and extended the lifetime of wind farms.
Process of Quantitative Risk Analysis
Dr. Wagner outlines the general steps involved in a quantitative risk analysis project. He emphasizes the importance of good communication with clients for understanding, gathering and interpretating the data.
Steps:
- Exploration: Collaborating with clients to gather necessary data.
- Model Development: Creating a model based on collected data.
- Model Calibration: Adjusting the model to align with real-world data.
- Iterative Review: Reviewing the model with clients for validation and credibility.
- Result Generation: Producing actionable recommendations based on the model.
Potential and Challenges
I. Potential
- Informed Decision-Making: Quantitative risk analysis offers the potential for making more informed decisions by understanding and preparing for various levels of risk, rather than aiming for absolute safety which doesn’t exist.
- Resource Optimization: It allows for the optimization of resources by directing efforts and investments toward mitigating risks that have the highest probability and potential impact, thereby preventing over-engineering and unnecessary expenses.
- Future-Proofing Infrastructure: By identifying the probability of rare but catastrophic events, quantitative risk analysis helps in the design and maintenance of infrastructure that can withstand such events, thus future-proofing investments.
II. Challenges
- Understanding of Probabilistic Concepts: Clients often struggle with the probabilistic nature of risk analysis, as it requires a shift from a deterministic mindset, which treats accidents as avoidable deterministic events, to a mindset that acknowledges their probabilistic nature and manages possible outcomes.
- Communication Barriers: Effective communication is crucial in explaining the value and implications of quantitative risk analysis to clients who may not be familiar with its principles and benefits.
- Data Sensitivity: Accurate risk analysis relies on detailed and sensitive data, which requires trust between the analyst and client for sharing and interpreting such information.
Conclusion
This paper summarizes a podcast episode on quantitative risk analysis, shedding light on its necessity for balancing safety with practicality in construction. It emphasizes the importance of understanding the probability of failures, the impossibility of absolute safety, and the need for a balanced approach. Aging infrastructure requires careful risk management to ensure continued functionality. The paper also covers case studies and outlines the risk analysis process, highlighting the benefits of informed decision-making and the challenges in communication and data management.
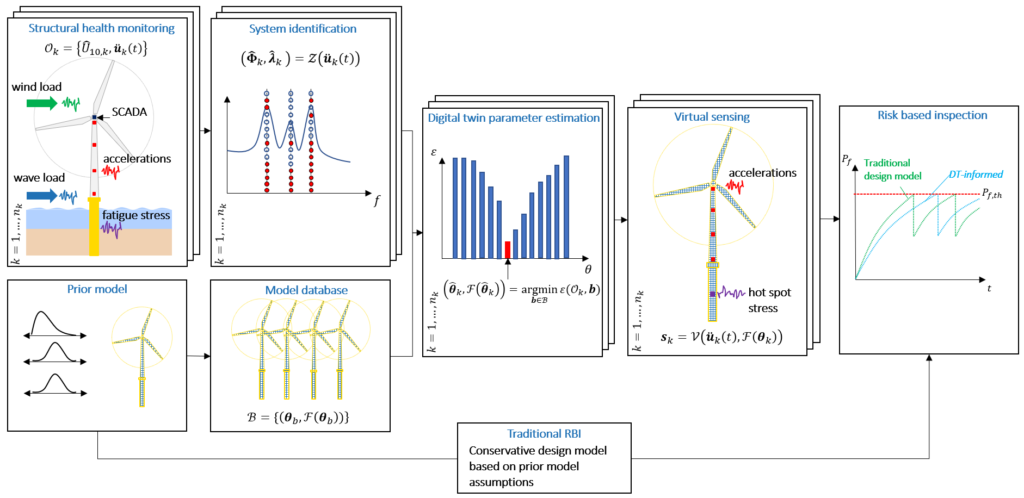
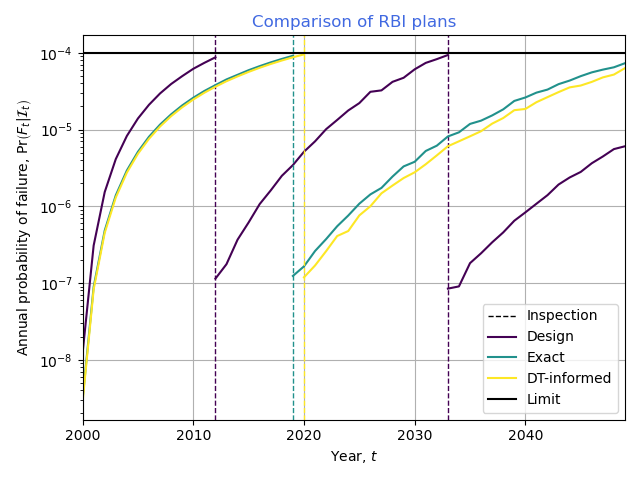
0 Comments